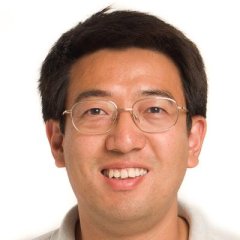
Registered user since Wed 12 Feb 2020
Contributions
View general profile
Registered user since Wed 12 Feb 2020
Contributions
Research (Full Papers)
Wed 14 Jun 2023 16:00 - 16:20 at Aurora Hall - Methodology and Secondary Studies Chair(s): Thomas FehlmannWith privacy concerns within the field of machine learning, federated learning (FL) was invented in 2017, in which the clients, such as mobile devices, compute or train a model and send the update to the centralized server. Choosing clients randomly for FL can harm learning performance due to different reasons. Many studies have proposed approaches to address the challenges of client selection of FL. However, there was no systematic literature review (SLR) on this topic. This SLR investigates the state of the art of client selection in FL and answers the challenges, solutions, and metrics to evaluate the solutions. We systematically reviewed 43 primary studies. The main challenges found in client selection are heterogeneity, resource allocation, communication costs, and fairness. The client selection schemes aim to improve the original random selection algorithm by focusing on one or more of the aforementioned challenges. The most common metric used is testing accuracy versus communication rounds, as testing accuracy measures the success- fulness of the learning and preferably in as few communication rounds as possible, as these are quite expensive. Although several possible improvements can be made with the current state of client selection, the most beneficial ones are evaluating the impact of unsuccessful clients and gaining a more theoretical understanding of the impact of fairness in federated learning.
DOI Authorizer link Pre-print File Attached