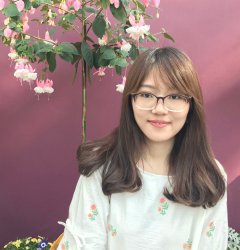
Registered user since Tue 29 Jan 2019
Contributions
Registered user since Tue 29 Jan 2019
Contributions
Research Papers
Tue 12 Sep 2023 15:54 - 16:06 at Plenary Room 2 - Code Generation 1 Chair(s): Kui LiuAutomated code generation has been extensively studied in recent literature. In this work, we first survey 66 participants to motivate a more pragmatic code generation scenario, i.e., library-oriented code generation, where the generated code should implement the functionally of the natural language query with the given library. We then revisit existing learning- based code generation techniques and find they have limited effectiveness in such a library-oriented code generation scenario. To address this limitation, we propose a novel library-oriented code generation technique, CodeGen4Libs, which incorporates two stages: import generation and code generation. The import generation stage generates import statements for the natural language query with the given third-party libraries, while the code generation stage generates concrete code based on the generated imports and the query. To evaluate the effectiveness of our approach, we conduct extensive experiments on a dataset of 403,780 data items. Our results demonstrate that CodeGen4Libs outperforms baseline models in both import generation and code generation stages, achieving improvements of up to 97.4% on EM (Exact Match), 54.5% on BLEU, and 53.5% on Hit@All. Overall, our proposed CodeGen4Libs approach shows promising results in generating high-quality code with specific third-party libraries, which can improve the efficiency and effectiveness of software development.
Pre-printResearch Papers
Wed 13 Sep 2023 14:30 - 14:42 at Plenary Room 2 - Code Summarization Chair(s): Ray Buseno description available
Research Papers
Thu 14 Sep 2023 16:21 - 16:34 at Room D - Configuration and Version Management Chair(s): Shahar MaozCollaborative development is critical to improve the productivity. Multiple contributors work simultaneously on the same project and might make changes to the same code locations. This can cause conflicts and require manual intervention from developers to resolve them. To alleviate the human efforts of manual conflict resolution, researchers have proposed various automatic techniques. More recently, deep learning models have been adopted to solve this problem and achieved state-of-the-art performance. However, these techniques leverage classification to combine the existing elements of input. The classification-based models cannot generate new tokens or produce flexible combinations, and have a wrong hypothesis that fine-grained conflicts of one single coarse-grained conflict are independent.
In this work, we propose to generate the resolutions of merge conflicts from a totally new perspective, that is, generation, and we present a conflict resolution technique, MergeGen. First, we design a structural and fine-grained conflict-aware representation for the merge conflicts. Then, we propose to leverage an encoder-decoder-based generative model to process the designed conflict representation and generate the resolutions auto-regressively. We further perform a comprehensive study to evaluate the effectiveness of MergeGen. The quantitative results show that MergeGen outperforms the state-of-the-art (SOTA) techniques from both precision and accuracy. Our evaluation on multiple programming languages verifies the good generalization ability of MergeGen. In addition, the ablation study shows that the major component of our technique makes a positive contribution to the performance of MergeGen, and the granularity analysis reveals the high tolerance of MergeGen to coarse-grained conflicts. Moreover, the analysis on generating new tokens further proves the advance of generative models.
Pre-print File Attached