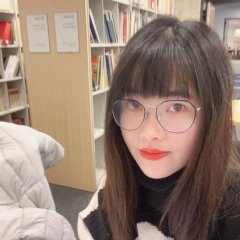
Registered user since Fri 4 Jun 2021
Contributions
Registered user since Fri 4 Jun 2021
Contributions
Journal-first Papers
Thu 14 Sep 2023 10:30 - 10:42 at Room D - Mobile Development 1 Chair(s): Jordan Samhino description available
File AttachedTemporal inconsistency in Android malware datasets can significantly distort the performance of these models, leading to inflated detection accuracy. Existing methods to detect temporal inconsistency in biased datasets, while useful, have limitations. They struggle when temporal inconsistencies are small, and their requirement of knowing the specific year of the dataset is often unfeasible in real-world scenarios. Motivated by these challenges, we introduce a novel and more effective method for identifying temporal inconsistency in Android malware datasets. Unlike prior studies, our method can identify the temporal inconsistency on an unknown dataset quickly and accurately without any assumption. Besides, We introduce a new dataset comprising 78k diverse Android samples, including malware and benign app samples spanning various time frames, specifically designed to study temporal inconsistency. Through a systematic evaluation of our proposed technique using this new dataset, we demonstrate its effectiveness in dealing with temporal inconsistency. Our experiments indicate that our method can achieve an accuracy rate of 98.3% in detecting temporal inconsistency in unknown datasets. Additionally, we established the efficacy of our feature selection process, which is integral to our approach, and demonstrated our method’s robustness when applied to unknown datasets. Our findings set a new benchmark in Android malware detection, paving the way for more reliable and accurate ML-based detection methods.