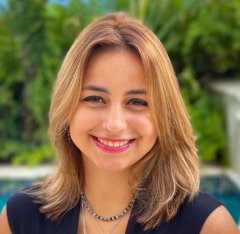
Registered user since Tue 15 Aug 2023
- Ph.D. student at The Graduate School and University Center of the City University of New York (CUNY).
- Member of the PONDER lab at Hunter College.
Contributions
Registered user since Tue 15 Aug 2023
Contributions
We aim to address a critical research problem regarding to the improvement of methodologies and tool-support systems for the comprehensive analysis and seamless transformation of imperative Deep Learning (DL) programs. DL frameworks have traditionally embraced deferred execution-style DL code. While scalable, such development tends to produce code that is error-prone. A more natural, less error-prone imperative DL frameworks encouraging eager execution have emerged at the expense of run-time performance. Though hybrid approaches aim for the “best of both worlds,” using them effectively requires subtle considerations to make code amenable to safe, accurate, and efficient graph execution— avoiding performance bottlenecks and semantically inequivalent results. Our proposed research tries to bridge this gap by comprehensively investigating scalable and reliable imperative DL programming, focusing in the development of novel methodologies and advanced tool-support mechanisms. We have initial work given where we analyze the challenges of migrating DL programs to graph execution and our progress to developing automated refactoring of imperative DL programs to graph execution.
- Ph.D. student at The Graduate School and University Center of the City University of New York (CUNY).
- Member of the PONDER lab at Hunter College.
Efficiency is essential to support responsiveness w.r.t. ever-growing datasets, especially for Deep Learning (DL) systems. DL frameworks have traditionally embraced deferred execution-style DL code—supporting symbolic, graph-based Deep Neural Network (DNN) computation. While scalable, such development is error-prone, non-intuitive, and difficult to debug. Consequently, more natural, imperative DL frameworks encouraging eager execution have emerged at the expense of run-time performance. Though hybrid approaches aim for the “best of both worlds,” using them effectively requires subtle considerations to make code amenable to safe, accurate, and efficient graph execution. We present our ongoing work on automated refactoring that assists developers in specifying whether and how their otherwise eagerly-executed imperative DL code could be reliably and efficiently executed as graphs while preserving semantics. The approach, based on a novel imperative tensor analysis, will automatically determine when it is safe and potentially advantageous to migrate imperative DL code to graph execution and modify decorator parameters or eagerly executing code already running as graphs. The approach is being implemented as a PyDev Eclipse IDE plug-in and uses the WALA Ariadne analysis framework. We discuss our ongoing work towards optimizing imperative DL code to its full potential.
Pre-print File Attached