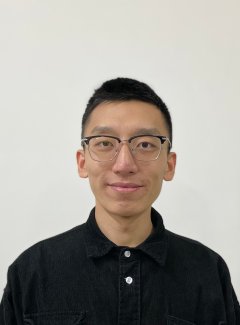
Registered user since Thu 17 Aug 2023
Contributions
Information retrieval-based fault localization (IRFL) techniques have been proposed as a solution to identify the files that are likely to contain faults that are root causes of failures reported by users. These techniques have been extensively studied to accurately rank source files, however, none of the existing approaches have focused on the specific case of concurrent programs. This is a critical issue since concurrency bugs are notoriously difficult to identify. To address this problem, this paper presents a novel approach called BLCoiR, which aims to reformulate bug report queries to more accurately localize source files related to concurrency bugs. The key idea of BLCoiR is based on a novel knowledge graph (KG), which represents the domain entities extracted from the concurrency bug reports and their semantic relations. The KG is then transformed into the IR query to perform fault localization. BLCoiR leverages natural language processing (NLP) and concept modeling techniques to construct the knowledge graph. Specifically, NLP techniques are used to extract relevant entities from the bug reports, such as the word entities related to concurrency constructs. These entities are then linked together based on their semantic relationships, forming the KG. We have conducted an empirical study on 692 concurrency bug reports from 44 real-world applications. The results show that BLCoiR outperforms existing IRFL techniques in terms of accuracy and efficiency in localizing concurrency bugs. BLCoiR demonstrates effectiveness of using a knowledge graph to model the domain entities and their relationships, providing a promising direction for future research in this area.
Pre-print