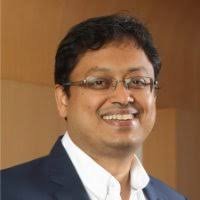
Registered user since Wed 27 Mar 2019
Contributions
Registered user since Wed 27 Mar 2019
Contributions
Industry Showcase (Papers)
Tue 12 Sep 2023 14:18 - 14:30 at Room D - Smart Contracts, Blockchain, Energy efficiency, and green softwareAdvances in technologies like artificial intelligence and metaverse have led to a proliferation of software systems in business and everyday life. With this widespread penetration, the carbon emissions of software are rapidly growing as well, thereby negatively impacting the long-term sustainability of our environment. Hence, optimizing software from a sustainability standpoint becomes more crucial than ever. We believe that the adoption of automated tools that can identify energy-inefficient patterns in the code and guide appropriate refactoring can significantly assist in this optimization. In this extended abstract, we present an industry case study that evaluates the sustainability impact of refactoring energy-inefficient code patterns identified by automated software sustainability assessment tools for a large application. Preliminary results highlight a positive impact on the application’s sustainability post-refactoring, leading to a 29% decrease in energy consumption.
NIER Track
Tue 12 Sep 2023 14:42 - 14:54 at Room D - Smart Contracts, Blockchain, Energy efficiency, and green softwareno description available
Industry Showcase (Papers)
Tue 12 Sep 2023 14:30 - 14:42 at Room D - Smart Contracts, Blockchain, Energy efficiency, and green softwareAs the world takes cognizance of AI’s growing role in greenhouse gas(GHG) and carbon emissions, the focus of AI research & development is shifting towards inclusion of energy efficiency as another core metric. Sustainability, a core agenda for most organizations, is also being viewed as a core nonfunctional requirement in software engineering. A similar effort is being undertaken to extend sustainability principles to AI-based systems with focus on energy efficient training and inference techniques. But an important question arises, does there even exist any metrics or methods which can quantify adoption of “green” practices in the life cycle of AI-based systems? There is a huge gap which exists between the growing research corpus related to sustainable practices in AI research and its adoption at an industry scale. The goal of this work is to introduce a methodology and novel metric for assessing ”greenness” of any AI-based system and its development process, based on energy efficient AI research and practices. The novel metric, termed as Green AI Quotient, would be a key step towards AI practitioner’s Green AI journey. Empirical validation of our approach suggest that Green AI Quotient is able to encourage adoption and raise awareness regarding sustainable practices in AI lifecycle.