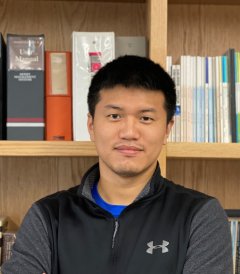
Registered user since Mon 28 Aug 2023
Contributions
Registered user since Mon 28 Aug 2023
Contributions
Tool Demonstrations
Thu 14 Sep 2023 13:42 - 13:54 at Room D - Mobile Development 2 Chair(s): Jordan SamhiAndroid applications are getting bigger with an increasing number of features. However, not all the features are needed by a specific user. The unnecessary features can increase the attack surface and cost additional resources (e.g., storage and memory). Therefore, it is important to remove unnecessary features from Android applications. However, it is difficult for the end users to fully explore the apps to identify the unnecessary features, and there is no off-the-shelf tool available to assist users to debloat the apps by themselves. In this work, we propose AutoDebloater to debloat Android applications automatically for end users. AutoDebloater is a web application that can be accessed by end-users through a web browser. In particular, AutoDebloater can automatically explore an app and identify the transitions between activities. Then, AutoDebloater will present the Activity Transition Graph to users and ask them to select the activities they do not want to keep. Finally, AutoDebloater will remove the activities that are selected by users from the app. We conducted a user study on five Android apps downloaded from three categories (i.e., Finance, Tools, and Navigation) in Google Play and F-Droid. The results show that users are satisfied with AutoDebloater in terms of the stability of the debloated apps and the ability of AutoDebloater to identify features that are never noticed before. The tool is available at http://autodebloater.club. The code is available at https://github.com/jiakun-liu/autodebloater/ and the demonstration video can be found at https://youtu.be/Gmz0-p2n9D4
Research Papers
Tue 12 Sep 2023 11:18 - 11:30 at Room C - Testing AI Systems 1 Chair(s): Leonardo MarianiLearning-based techniques, especially advanced Large Language Models (LLMs) for code, have gained considerable popularity in various software engineering (SE) tasks. However, most existing works focus on designing better learning-based models and pay less attention to the properties of datasets. Learning-based models, including popular LLMs for code, heavily rely on data, and the data’s properties (e.g., data distribution) could significantly affect their behavior. We conducted an exploratory study on the distribution of SE data and found that such data usually follows a skewed distribution (i.e., long-tailed distribution) where a small number of classes have an extensive collection of samples, while a large number of classes have very few samples. We investigate three distinct SE tasks and analyze the impacts of long-tailed distribution on the performance of LLMs for code. Our experimental results reveal that the long-tailed distribution has a substantial impact on the effectiveness of LLMs for code. Specifically, LLMs for code perform between 30.0% and 254.0% worse on data samples associated with infrequent labels compared to data samples of frequent labels. Our study provides a better understanding of the effects of long-tailed distributions on popular LLMs for code and insights for the future development of SE automation.
Pre-printno description available