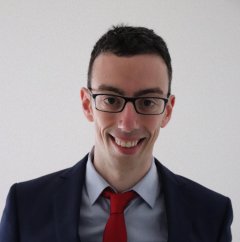
Registered user since Tue 20 Feb 2018
Contributions
Registered user since Tue 20 Feb 2018
Contributions
Research Papers
Wed 13 Sep 2023 11:06 - 11:18 at Plenary Room 2 - Code Quality and Code Smells Chair(s): Bernd FischerUpon evolving their software, organizations and individual developers have to spend a substantial effort to pay back technical debt, i.e., the fact that software is released in a shape not as good as it should be, e.g., in terms of functionality, reliability, or maintainability. This paper empirically investigates the extent to which technical debt can be automatically paid back by neural-based generative models, and in particular models exploiting different strategies for pre-training and fine-tuning. We start by extracting a dateset of 5,039 Self-Admitted Technical Debt (SATD) removals from 595 open-source projects. SATD refers to technical debt instances documented (e.g., via code comments) by developers. We use this dataset to experiment with seven different generative deep learning (DL) model configurations. Specifically, we compare transformers pre-trained and fine-tuned with different combinations of training objectives, including the fixing of generic code changes, SATD removals, and SATD-comment prompt tuning. Also, we investigate the applicability in this context of a recently-available Large Language Model (LLM)-based chat bot. Results of our study indicate that the automated repayment of SATD is a challenging task, with the best model we experimented with able to automatically fix ~2% to 8% of test instances, depending on the number of attempts it is allowed to make. Given the limited size of the fine-tuning dataset (~5k instances), the model’s pre-training plays a fundamental role in boosting performance. Also, the ability to remove SATD steadily drops if the comment documenting the SATD is not provided as input to the model. Finally, we found general-purpose LLMs to not be a competitive approach for addressing SATD.
Pre-print File AttachedJournal-first Papers
Tue 12 Sep 2023 11:30 - 11:42 at Room D - Infrastructure, Build, and Logs Chair(s): Fatemeh Hendijani Fard, Arie van DeursenDocker is a containerization technology that allows developers to ship software applications along with their dependencies in Docker images. Developers can extend existing images using them as base images when writing Dockerfiles. However, a lot of alternative functionally-equivalent base images are available. While many studies define and evaluate quality features that can be extracted from Docker artifacts, it is still unclear what are the criteria on which developers choose a base image over another. In this paper, we aim to fill this gap. First, we conduct a literature review through which we define a taxonomy of quality features, identifying two main groups: Configuration-related features (i.e., mainly related to the Dockerfile and image build process), and externally observable features (i.e., what the Docker image users can observe). Second, we ran an empirical study considering the developers’ preference for 2,441 Docker images in 1,911 open-source software projects. We want to understand (i) how the externally observable features influence the developers’ preferences, and (ii) how they are related to the configuration-related features. Our results pave the way to the definition of a reliable quality measure for Docker artifacts, along with tools that support developers for a quality-aware development of them
Link to publication