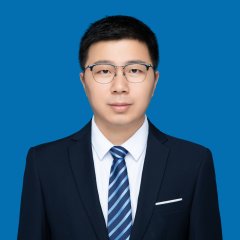
Registered user since Wed 17 Mar 2021
Contributions
View general profile
Registered user since Wed 17 Mar 2021
Contributions
Research Papers
Thu 13 Oct 2022 11:10 - 11:30 at Ballroom C East - Technical Session 23 - Security Chair(s): John-Paul OreSmart contracts have been widely and rapidly used to automate financial and business transactions together with blockchains, helping people make agreements while minimizing trusts. With millions of smart contracts deployed on blockchain, various bugs and vulnerabilities in smart contracts have emerged. Following the rapid development of deep learning, many recent studies have used deep learning for vulnerability detection to conduct security checks before deploying smart contracts. However, these approaches are limited to providing only the decision on whether a smart contract is vulnerable or not, without further analysis on locating suspicious statements potentially responsible for the detected vulnerability.
To address this problem, we propose a deep learning based two-phase smart contract debugger for the Reentrancy vulnerability, one of the most severe vulnerabilities, named as ReVulDL: Reentrancy Vulnerability Detection and Localization. ReVulDL integrates the vulnerability detection and localization into a unified debugging pipeline. For the detection phase, given a smart contract, ReVulDL uses a graph-based pre-training model to learn the complex relationships in propagation chains for detecting whether the smart contract contains a reentrancy vulnerability. For the localization phase, if a reentrancy vulnerability is detected, ReVulDL utilizes interpretable machine learning to locate the suspicious statements in smart contract to provide interpretations of the detected vulnerability. Our large-scale empirical study on 47,398 smart contracts shows that ReVulDL achieves promising results in detecting reentrancy vulnerabilities (e.g., outperforming 15 state-of-the-art vulnerability detection approaches) and locating vulnerable statements (e.g., 70.38% of the vulnerable statements are ranked within top-10).
Research Papers
Thu 13 Oct 2022 14:20 - 14:40 at Ballroom C East - Technical Session 25 - Software Repairs Chair(s): Yannic NollerAutomated program repair (APR) holds the promise of aiding manual debugging activities. Over a decade of evolution, a broad range of APR techniques have been proposed and evaluated on a set of real-world bug datasets. However, while more and more bugs have been correctly fixed, we observe that the growth of newly fixed bugs by APR techniques has hit a bottleneck in recent years. In this work, we explore the possibility of addressing complicated bugs by proposing TransplantFix, a novel APR technique that leverages graph differencing-based transplantation from the donor method. The key novelty of TransplantFix lies in three aspects: 1) we propose to use a graph-based differencing algorithm to distill semantic fix actions from the donor method; 2) we devise an inheritance-hierarchy-aware code search approach to identify donor methods with similar functionality; 3) we present a namespace transfer approach to effectively adapt donor code.
We investigate the unique contributions of TransplantFix by conducting an extensive comparison that covers a total of 42 APR techniques and evaluating TransplantFix on 839 real-world bugs from Defects4J v1.2 and v2.0. TransplantFix presents superior results in three aspects. First, it has achieved the best performance as compared to the state-of-the-art APR techniques proposed in the last three years, in terms of the number of newly fixed bugs, reaching a 60%-300% improvement. Furthermore, not relying on any fix actions crafted manually or learned from big data, it reaches the best generalizability among all APR techniques evaluated on Defects4J v1.2 and v2.0. In addition, it shows the potential to synthesize complicated patches consisting of at most eight-line insertions at a hunk. TransplantFix presents fresh insights and a promising avenue for follow-up research towards addressing more complicated bugs.