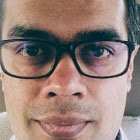
Registered user since Sat 14 Apr 2018
Contributions
View general profile
Registered user since Sat 14 Apr 2018
Contributions
Late Breaking Results
Wed 12 Oct 2022 17:30 - 17:40 at Gold A - Technical Session 20 - Web, Cloud, Networking Chair(s): Karine Even-MendozaSustainable software engineering has received a lot of attention in recent times, as we witness an ever-growing slice of energy use, for example, at data centers, as software systems utilize the underlying infrastructure. Characterizing servers for their energy use accurately without being intrusive, is therefore important to make sustainable software deployment choices. In this paper, we introduce ESAVE which is a machine learning-based approach that leverages a small set of hardware attributes to characterize any server or virtual machine’s energy use across different levels of utilization. This is based upon an extensive exploration of multiple ML approaches, with a focus on a minimal set of required attributes, while showcasing good accuracy. Early validations show that ESAVE has only around 12% average prediction error. This approach is non-intrusive and therefore can enable many sustainable software engineering use cases, promoting greener DevOps.
Industry Showcase
Wed 12 Oct 2022 17:40 - 18:00 at Gold A - Technical Session 20 - Web, Cloud, Networking Chair(s): Karine Even-MendozaDeploying applications on hybrid clouds with computational artifacts distributed over public backends and private edges involve several constraints. Designing such deployment requires application architects to solve several challenges, spanning over hard regulatory policy constraints as well as business policy constraints such as enablement of privacy by on-prem processing of data to the extent the business wants, backend support of privacy enabling technologies (PET), sustainability in terms of green energy utilization, latency sensitivity of the application. In this paper, we propose to optimize hybrid cloud application architectures, while taking all those factors into consideration, and empirically demonstrate the effectiveness of our approach. To the best of our knowledge, this work is the first of its kind.