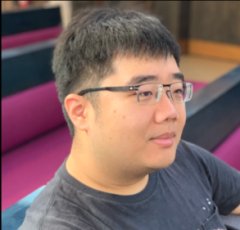
Registered user since Thu 26 Oct 2017
Assistant professor at Concordia University.
Contributions
View general profile
Registered user since Thu 26 Oct 2017
Assistant professor at Concordia University.
Contributions
Research Papers
Wed 12 Oct 2022 13:30 - 13:50 at Banquet A - Technical Session 14 - Bug Prediction and Localization Chair(s): David LoContinuous integration (CI) is a software practice by which developers frequently merge and test code under development. In CI settings, the change information is finer-grained. Prior studies have widely studied and evaluated the performance of spectrum-based fault localization (SBFL) techniques. While the continuous nature of CI requires the code changes to be atomic and presents fine-grained information on what part of the system is being changed, traditional SBFL techniques do not benefit from it. In this paper, we conduct an empirical study on the effectiveness of using and integrating code and coverage changes for fault localization in CI settings. We conduct our study on seven open source systems, with a total of 192 faults. We find that while both change information covers a reduced search space compared to code coverage, the percentages of faulty methods in the search space are 7 and 14 times higher than code coverage for code changes and coverage changes, respectively. Then, we propose three change-based fault localization techniques and compare them with Ochiai, a commonly used SBFL technique. Our results show that all three change-based techniques outperform Ochiai, achieving an improvement that varies from 7% to 23% and 17% to 24% over Ochiai for average MAP and MRR, respectively. Moreover, we find that our change-based fault localization techniques can be integrated with Ochiai, achieving up to 53% and 52% improvement over Ochiai in average MAP and MRR respectively, and locating 41 more faults at Top-1.
Journal-first Papers
Wed 12 Oct 2022 16:50 - 17:10 at Gold A - Technical Session 20 - Web, Cloud, Networking Chair(s): Karine Even-MendozaIn industrial environments it is critical to find out the capacity of a system and plan for a deployment layout that meets the production traffic demands. The system capacity is influenced by both the performance of the system’s constituting components and the physical environment setup. In a large system, the configuration parameters of individual components give the flexibility to developers and load test engineers to tune system performance without changing the source code. However, due to the large search space, estimating the capacity of the system given different configuration values is a challenging and costly process. In this paper, we propose an approach, called MLASP, that uses machine learning models to predict the system key performance indicators (i.e., KPIs), such as throughput, given a set of features made off configuration parameter values, including server cluster setup, to help engineers in capacity planning for production environments. Under the same load, we evaluate MLASP on two large-scale mission-critical enterprise systems developed by Ericsson and on one open-source system. We find that: 1) MLASP can predict the system throughput with a very high accuracy. The difference between the predicted and the actual throughput is less than 1%; and 2) By using only a small subset of the training data (e.g., 3% of the entire data for the open-source system), MLASP can still predict the throughput accurately. We also document our experience of successfully integrating the approach into an industrial setting. In summary, this paper highlights the benefits and potential of using machine learning models to assist load test engineers in capacity planning.
Link to publication DOI