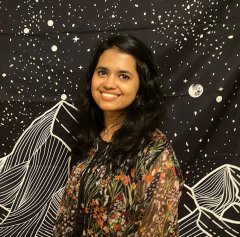
Registered user since Fri 19 Feb 2021
Contributions
View general profile
Registered user since Fri 19 Feb 2021
Contributions
Being extremely dominated by men, software development organizations lack diversity. People from other groups often encounter sexist, misogynistic, and discriminatory (SMD) speech during communication. To identify SMD contents, I aim to build an automatic misogyny identification (AMI) tool for the domain of software developers. On this goal, I built a dataset of 10,138 pull request comments mined from Github based on a keyword-based selection, followed by manual validation. Using ten-fold cross-validation, I evaluated ten machine learning algorithms for automatic identification. The best performing model achieved 67.7% precision, 55.9% recall, 60.9% f-score, and 96.2% accuracy for the best performing model.
Doctoral Symposium
Mon 10 Oct 2022 14:00 - 14:30 at Ambassador A - Session 3 - Human Factors & TestingContemporary software development organizations are dominated by straight males and lack diversity. As a result, people from other demographic such as women and LGBTQ+ often encounter bias, sexism, and misogyny. Due to negative experiences, many women switch careers. Therefore, biases pose barriers to promote diversity and inclusion. To get benefits from diverse pools of talents and reduce the attrition rate of minorities, we need to identify the degree and effect of various biases and develop mitigation strategies. Therefore, my dissertation study aims at promoting diversity and inclusion among software development organizations by identifying the manifestation, magnitude, and frequency of various gender biases. For this purpose, I plan to investigate i) the effect of gender of the contributors in the code review process of Free/Libre Open Source Software (FLOSS) projects, ii) the frequency of different dimensions of gender bias and their effect, and iii) develop a tool to identify sexist and misogynistic and derogatory (SMD) texts.