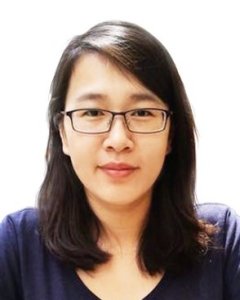
Registered user since Tue 23 Nov 2021
Contributions
View general profile
Registered user since Tue 23 Nov 2021
Contributions
Research Papers
Wed 12 Oct 2022 11:20 - 11:40 at Gold A - Technical Session 11 - Analysis and Types Chair(s): Thiago FerreiraPartial code usually involves non-fully-qualified type names (non-FQNs) and undeclared receiving objects. Resolving the FQNs of these non-FQN types and undeclared receiving objects (referred to as type inference) is the prerequisite to effective search and reuse of partial code. Existing dictionary-lookup based methods build a symbolic knowledge base of API names and code contexts, which involve significant compilation overhead and are sensitive to unseen API names and code context variations. In this paper, we formulate type inference as a cloze-style fill-in-blank language task. Built on source code naturalness, our approach trains a code masked language model (MLM) as a neural knowledge base of code elements with a novel ``pre-train, prompt and predict'' paradigm from raw source code. Our approach is lightweight and has minimum requirements on code compilation. Unlike existing symbolic name and context matching for type inference, our prompt-tuned code MLM packs FQN syntax and usage in its parameters and supports fuzzy neural type inference. We systematically evaluate our approach on a large amount of source code from GitHub and Stack Overflow. Our results confirm the effectiveness of our approach design and the practicality for partial code type inference. As the first of its kind, our neural type inference method opens the door to many innovative ways of using partial code.
Research Papers
Thu 13 Oct 2022 10:00 - 10:20 at Gold A - Technical Session 24 - Human Aspects Chair(s): Silvia AbrahãoExploratory testing is an effective testing approach which leverages the tester’s knowledge and creativity to design test cases to provoke and recognize failures at the system level from the end user’s perspective. Although some principles and guidelines have been proposed to guide exploratory testing, there are no effective tools for automatic generation of exploratory test scenarios (a.k.a soap opera tests). Existing test generation techniques rely on specifications, program differences and fuzzing, which are not suitable for exploratory test generation. In this paper, we propose to leverages the scenario and oracle knowledge in bug reports to generate soap opera test scenarios. We develop open information extraction methods to construct a system knowledge graph (KG) of user tasks and failures from the steps to reproduce, expected results and observed results in bug reports. We construct a proof-of-concept KG from 25,939 bugs of the Firefox browser. Our evaluation shows the constructed KG is of high quality. Based on the KG, we creates soap opera test scenarios by combining the scenarios of relevant bugs, and develop a web tool to present the created test scenarios and support exploratory testing. In our user study, 5 users find 18 bugs from 5 seed bugs in 2 hours using our tool, while the control group find only 5 bugs based on the recommended similar bugs.