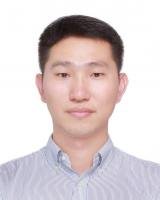
Registered user since Sun 4 Sep 2022
Contributions
View general profile
Registered user since Sun 4 Sep 2022
Contributions
Research Papers
Wed 12 Oct 2022 17:10 - 17:30 at Gold A - Technical Session 20 - Web, Cloud, Networking Chair(s): Karine Even-MendozaWith the ever increasing scale and complexity of online systems, incidents are gradually becoming commonplace. Without appropriate handling, they can seriously harm the system availability. However, in large-scale online systems, these incidents are usually drowning in a slew of issues (i.e., something abnormal, while not necessarily an incident), rendering them difficult to handle. Typically, these issues will result in a cascading effect across the system, and a proper management of the incidents depends heavily on a thorough analysis of this effect. Therefore, in this paper, we propose a method to automatically analyze the cascading effect of availability issues in online systems and extract the corresponding graph based issue representations incorporating both of the issue symptoms and affected service attributes. With the extracted representations, we train and utilize a graph neural networks based model to perform incident detection. Then, for the detected incident, we leverage the PageRank algorithm with a flexible transition matrix design to locate its root cause. We evaluate our approach using real-world data collected from a very large instant messaging company. The results confirm the effectiveness of our approach. Moreover, our approach is successfully deployed in the company and eases the burden of operators in the face of a flood of issues and related alert signals.
Research Papers
Thu 13 Oct 2022 17:00 - 17:20 at Ballroom C East - Technical Session 29 - AI for SE II Chair(s): Tim MenziesMany real-world online systems require the forecast of monitored time series metrics to detect and localize anomalies, schedule resources, and assist relevant staffs in decision making. Even though many time series forecasting techniques have been proposed, few of them can be directly applied in online systems due to their efficiency and lack of model sharing. To address the challenges, this paper presents TTSF-transformer, a transferable time series forecasting service using deep transformer model. TTSF-transformer normalizes multiple metric frequencies to ensure the model sharing across multi-source systems, employs a deep transformer model with Bayesian estimation to generate the predictive marginal distribution, and introduces transfer learning and incremental learning into the training process to ensure the long-term performance. We conduct experiments on real-world time series metrics from two different types of game business in Tencent. The results show that TTSF-transformer significantly outperforms other state-of-the-art methods and is suitable for wide deployment in large online systems.