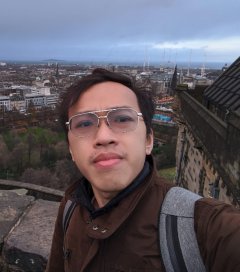
Registered user since Thu 25 Jan 2018
Contributions
View general profile
Registered user since Thu 25 Jan 2018
Contributions
Late Breaking Results
Thu 13 Oct 2022 10:50 - 11:00 at Ballroom C East - Technical Session 23 - Security Chair(s): John-Paul OreExisting approaches to improving the robustness of source code models concentrate on recognizing adversarial samples rather than valid samples that fall outside of a given distribution, which we refer to as out-of-distribution (OOD) samples. Recognizing such OOD samples is the novel problem investigated in this paper. To this end, we propose to use an auxiliary dataset (out-of-distribution) such that, when trained together with the main dataset, they will enhance the model’s robustness. We adapt energy-bounded learning objective function to assign a higher score to in-distribution samples and a lower score to out-of-distribution samples in order to incorporate such out-of-distribution samples into the training process of source code models. In terms of OOD detection and adversarial samples detection, our evaluation results demonstrate a greater robustness for existing source code models to become more accurate at recognizing OOD data while being more resistant to adversarial attacks at the same time.
Late Breaking Results
Wed 12 Oct 2022 17:40 - 17:50 at Banquet B - Technical Session 17 - SE for AI Chair(s): Tim MenziesDespite the recent trend of developing and applying neural source code models to software engineering tasks, the quality of such models is insufficient for real-world use. This is because there could be noise in the source code corpora used to train such models. We adapt data-influence methods to detect such noises in this paper. Data-influence methods are used in machine learning to evaluate the similarity of a target sample to the correct samples in order to determine whether or not the target sample is noisy. Our evaluation results show that data-influence methods can identify noisy samples from neural code models in classification-based tasks. We anticipate that this approach will contribute to the larger vision of developing better neural source code models from a data-centric perspective, which is a key driver for developing useful source code models in practice.