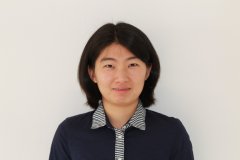
Registered user since Fri 16 Jan 2015
Contributions
View general profile
Registered user since Fri 16 Jan 2015
Contributions
Journal-first Papers
Thu 13 Oct 2022 10:10 - 10:30 at Ballroom C East - Technical Session 23 - Security Chair(s): John-Paul OreThe Java platform provides various cryptographic APIs to facilitate secure coding. However, correctly using these APIs is challenging for developers who lack cybersecurity training. Prior work shows that many developers misused APIs and consequently introduced vulnerabilities into their software. To eliminate such vulnerabilities, people created tools to detect and/or fix cryptographic API misuses. However, it is still unknown (1) how current tools are designed to detect cryptographic API misuses, (2) how effectively the tools work to locate API misuses, and (3) how developers perceive the usefulness of tools’ outputs. For this paper, we conducted an empirical study to investigate the research questions mentioned above. Specifically, we first conducted a literature survey on existing tools and compared their approach design from different angles. Then we applied six of the tools to three popularly used benchmarks to measure tools’ effectiveness of API-misuse detection. Next, we applied the tools to 200 Apache projects and sent 57 vulnerability reports to developers for their feedback. Our study revealed interesting phenomena. For instance, none of the six tools was found universally better than the others; however, CogniCrypt, CogniGuard, and Xanitizer outperformed SonarQube. More developers rejected tools’ reports than those who accepted reports (30 vs. 9) due to their concerns on tools’ capabilities, the correctness of suggested fixes, and the exploitability of reported issues. This study reveals a significant gap between the state-of-the-art tools and developers’ expectations; it sheds light on future research in vulnerability detection.
DOI Pre-printResearch Papers
Wed 12 Oct 2022 10:40 - 11:00 at Banquet B - Technical Session 12 - Builds and Versions Chair(s): Yi LiDuring software merge, the edits from different branches can textually overlap (i.e., textual conflicts) or cause build and test errors (i.e., build and test conflicts), jeopardizing programmer productivity and software quality. Existing tools primarily focus on textual conflicts; few tools detect higher-order conflicts (i.e., build and test conflicts). However, existing detectors of build conflicts are quite limited. Due to their heavy usage of automatic build, the build-based detectors (e.g., Crystal) can only report build errors instead of pinpointing the root causes; developers have to manually locate conflicting edits. Furthermore, these detectors only help when the branches-to-merge have no textual conflict.
We present a novel approach Bucond (“build conflict detector”) to detect conflicts via static analysis, instead of using automatic build. Given the three program versions in a merging scenario: base 𝑏, left 𝑙 , and right 𝑟 , Bucond first models each version as a graph, and compares graphs to extract entity-related edits (e.g., rename a class) in 𝑙 and 𝑟 branches. Our insight is that build conflicts occur when certain edits are co-applied to related entities between branches. Bucond realizes this insight via pattern-based reasoning, to identify any cross-branch edit combination that can trigger build conflicts (e.g., one branch adds a reference to field F while the other branch removes F). We conducted a systematic exploration to devise 57 conflicting-edit patterns, covering 97% of total build conflicts in our experiments. We evaluated Bucond with three datasets and Bucond worked effectively on all datasets. It complements existing build-based conflict detectors, as it (1) detects conflicts with 100% precision and 88%–100% recall, (2) pinpoints the conflicting edits, and (3) works well when those detectors are inapplicable.