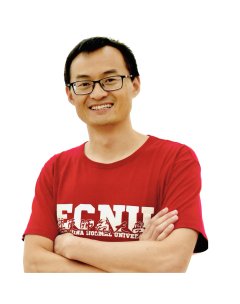
Registered user since Tue 18 Apr 2017
Contributions
View general profile
Registered user since Tue 18 Apr 2017
Contributions
Research Papers
Thu 13 Oct 2022 17:30 - 17:50 at Banquet B - Technical Session 32 - Formal Methods and Models II Chair(s): Khouloud GaaloulDeep learning has become a promising programming paradigm in software development, owing to its surprising performance in solving many challenging tasks. Deep neural networks (DNNs) are increasingly being deployed in practice, but are limited on resource-constrained devices owing to their demand for computational power. Quantization has emerged as a promising technique to reduce the size of DNNs with comparable accuracy as their floating-point numbered counterparts. The resulting quantized neural networks (QNNs) can be implemented energy-efficiently. Similar to their floating-point numbered counterparts, quality assurance techniques for QNNs, such as testing and formal verification, are essential but are currently less explored. In this work, we propose a novel and efficient formal verification approach for QNNs. In particular, we are the first to propose an encoding that reduces the verification problem of QNNs into the solving of integer linear constraints, which can be solved using off-of-the-shelf solvers. Our encoding is both sound and complete. We demonstrate the application of our approach on local robustness verification and maximum robustness radius computation. We implement our approach in a prototype tool QVIP and conduct a thorough evaluation. Experimental results on QNNs with different quantization bits confirm the effectiveness and efficiency of our approach, e.g., our approach is two orders of magnitude faster and able to solve more verification tasks in the same time limit than the state-of-the-art methods.
Research Papers
Wed 12 Oct 2022 16:40 - 17:00 at Ballroom C East - Technical Session 19 - Formal Methods and Models I Chair(s): Michalis FamelisThe robustness of deep neural networks is crucial to modern AI-enabled systems. Formal verification has been demonstrated effective in providing certified robustness guarantees. Sigmoid-like neural networks have been adopted in a wide range of applications. Due to their non-linearity, Sigmoid-like activation functions are usually over-approximated for efficient verification, which inevitably introduces imprecision. Considerable efforts have been devoted to finding the so-called tighter approximations to obtain more precise verification results. However, existing tightness definitions are heuristic and lack a theoretical foundation. We conduct a thorough empirical analysis of existing neuron-wise characterizations of tightness and reveal that they are superior only on specific neural networks. We then introduce the notion of network-wise tightness as a unified tightness definition and show that computing network-wise tightness is a complex non-convex optimization problem. We bypass the complexity from different perspectives via two efficient, provably tightest approximations. The experimental results demonstrate the promising performance achievement of our approaches over state of the art: (i) achieving up to 436.36% improvement to certified lower robustness bounds; and (ii) exhibiting notably more precise verification results on convolutional networks.