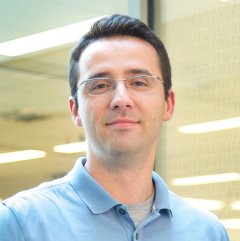
Registered user since Sun 2 Aug 2020
Contributions
View general profile
Registered user since Sun 2 Aug 2020
Contributions
Research Papers
Thu 13 Oct 2022 10:20 - 10:40 at Gold A - Technical Session 24 - Human Aspects Chair(s): Silvia AbrahãoEmotions (e.g., Joy, Anger) are prevalent in daily software engineering (SE) activities, and are known to be significant indicators of work productivity (e.g., bug fixing efficiency). Recent studies have shown that directly applying general purpose emotion classification tools to SE corpora is not effective. Even within the SE domain, tool performance degrades significantly when trained on one communication channel and evaluated on another (e.g, StackOverflow vs. GitHub comments). Retraining a tool with channel-specific data takes significant effort since manually annotating large datasets of ground truth data is expensive.
In this paper, we address this data scarcity problem by automatically creating new training data using a data augmentation technique. Based on an analysis of the types of errors made by popular SE-specific emotion recognition tools, we specifically target our data augmentation strategy in order to improve the performance of emotion recognition. Our results show an average improvement of 9.3% in micro F1-Score for three existing emotion classification tools (ESEM-E, EMTk, SEntiMoji) when trained with our best augmentation strategy.
Pre-printJournal-first Papers
Thu 13 Oct 2022 11:30 - 11:50 at Gold A - Technical Session 24 - Human Aspects Chair(s): Silvia AbrahãoSoftware engineers are crowdsourcing answers to their everyday challenges on Q&A forums (e.g., Stack Overflow) and more recently in public chat communities such as Slack, IRC and Gitter. Many software-related chat conversations contain valuable expert knowledge that is useful for both mining to improve programming support tools and for readers who did not participate in the original chat conversations. However, most chat platforms and communities do not contain built-in quality indicators (e.g., accepted answers, vote counts). Therefore, it is difficult to identify conversations that contain useful information for mining or reading, i.e,. conversations of post hoc quality. In this paper, we investigate automatically detecting developer conversations of post hoc quality from public chat channels. We first describe an analysis of 400 developer conversations that indicate potential characteristics of post hoc quality, followed by a machine learning-based approach for automatically identifying conversations of post hoc quality. Our evaluation of 2000 annotated Slack conversations in four programming communities (python, clojure, elm, and racket) indicates that our approach can achieve precision of 0.82, recall of 0.90, F-measure of 0.86, and MCC of 0.57. To our knowledge, this is the first automated technique for detecting developer conversations of post hoc quality.
Link to publication