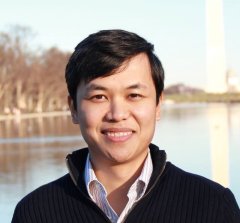
Registered user since Thu 7 Jun 2018
Contributions
View general profile
Registered user since Thu 7 Jun 2018
Contributions
NIER Track
Tue 11 Oct 2022 14:40 - 14:50 at Banquet A - Technical Session 6 - Source Code Manipulation Chair(s): Collin McMillanCode completion is an important feature in an IDE to improve developers’ productivity. Existing code completion approaches focus on completing the current code token, next token or statement, or code pattern. We propose AstCC, a code completion approach to suggest the next syntactic unit via an AST-based statistical language model. AstCC learns from a large code corpus to derive the next AST subtree representing a syntactic unit, and then fills in the template with the concrete variables from the current program scope. Our empirical evaluation shows that AstCC can correctly suggest the next syntactic unit in 33% of the cases, and in 62% of the cases, it correctly suggests within five candidates. We will also explain the potential applications of AstCC in automated program repair, automated test case generation, and syntactic pattern mining.
Research Papers
Wed 12 Oct 2022 13:30 - 13:50 at Ballroom C East - Technical Session 13 - Application Domains Chair(s): Andrea StoccoAutomatically producing behavioral exception (BE) API documentation helps developers correctly use the libraries. The state-of-the-art approaches are either rule-based, which is too restrictive in its applicability, or deep learning (DL)-based, which requires large training dataset. To address those issues, we propose StatGen, a novel hybrid approach between statistical machine translation (SMT) and tree-structured translation to generate BE documentation for any code and vice versa. We consider an API method to possess two levels of abstraction: the source code for the API method, and its documentation. StatGen is specifically designed for this two-way inference, taking advantages of the structures of source code and documentation to achieve higher accuracy. For practical use, if the code does not have BE documentation, StatGen can help users in writing it, and if it exists, one can use StatGen to verify the consistency between BE documentation and implementations. Moreover, it can generate BE code from existing BE documentation.
We conducted empirical experiments to intrinsically evaluate StatGen. We show that it achieves high precision (82% and 79%), and recall (86% and 90%), in inferring BE documentation from source code and vice versa. Our results show that StatGen achieves high accuracy in precision, recall, and BLEU score, and outperforms the state-of-the-art baselines in SMT, Neural Machine Translation, tree-based transformer, and dual-task learner. We showed StatGen’s usefulness in two applications. First, we used StatGen to generate the BE documentation for Apache APIs that lack of documentation by learning from the documentation of the equivalent APIs in JDK. 46% of the generated documentation were rated as useful and 41% as somewhat useful. In the second application, we used StatGen to detect the inconsistency between BE documentation and corresponding implementations of several packages in JDK8.