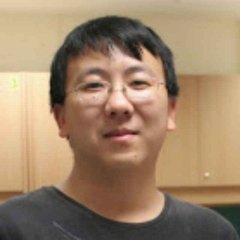
Registered user since Sun 30 May 2021
Contributions
View general profile
Registered user since Sun 30 May 2021
Contributions
Research Papers
Wed 12 Oct 2022 11:10 - 11:30 at Banquet A - Technical Session 10 - Testing I Chair(s): Gordon FraserVirtual personal assistant (VPA) services, e.g. Amazon Alexa and Google Assistant, are becoming increasingly popular recently. Users interact with them through voice-based apps, e.g. Amazon Alexa skills and Google Assistant actions. Unlike the desktop and mobile apps which have visible and intuitive graphical user interface (GUI) to facilitate interaction, VPA apps convey information purely verbally through the voice user interface (VUI), which is known to be limited in its invisibility, single mode and high demand of user attention. This may lead to various problems on the usability and correctness of VPA apps.
In this work, we propose a model-based framework named Vitas to handle VUI testing of VPA apps. Vitas interacts with the app VUI, and during the testing process, it retrieves semantic information from voice feedbacks by natural language processing. It incrementally constructs the finite state machine (FSM) model of the app with a weighted exploration strategy guided by key factors such as the coverage of app functionality. We conduct a large-scale testing on 41,581 VPA apps (i.e., skills) of Amazon Alexa, the most popular VPA service, and find that 51.29% of them have weaknesses. They largely suffer from problems such as unexpected exit/start, privacy violation and so on. Our work reveals the immaturity of the VUI designs and implementations in VPA apps, and sheds light on the improvement of several crucial aspects of VPA apps.
This submission includes the artifacts of our paper named \emph{Scrutinizing Privacy Policy Compliance of Virtual Personal Assistant Apps}.
DOIResearch Papers
Wed 12 Oct 2022 11:40 - 12:00 at Ballroom C East - Technical Session 9 - Security and Privacy Chair(s): Wei YangBrowser extensions have emerged as integrated characteristics in modern browsers, with the aim to boost the online browsing experience. Their advantageous position between a user and the Internet grants them easy access to the user’s sensitive personal data, which has raised mounting privacy concerns from both legislators and the extension users. In this work, we propose an end-to-end automatic extension privacy compliance auditing approach, analyzing the compliance of privacy policy versus regulation requirements and their actual privacy-related practices during runtime.
Our approach utilizes the state-of-the-art language processing model BERT for annotating the policy texts, and a hybrid technique to analyze the privacy-related elements (e.g., API calls and HTML objects) from the static source code and dynamically generated files during runtime. We collect a comprehensive dataset within 42 hours in April 2022, containing a total of 64,114 extensions. To facilitate the model training, we construct a corpus named PrivAud-100 which contains 100 manually annotated privacy policies. Based on this dataset and the corpus, we conduct a systematic audition, and identify widespread privacy compliance issues. We find around 92% of the extensions have at least one violation in either their privacy policies or data collection practices. We further propose an index to facilitate the filtering and identification of extensions with significant probability of privacy compliance violations. Our work should raise the awareness from the extension users, service providers, and platform operators, and encourage them to implement solutions towards better privacy compliance. To facilitate future research in this area, we have released our dataset.
Research Papers
Wed 12 Oct 2022 11:00 - 11:20 at Ballroom C East - Technical Session 9 - Security and Privacy Chair(s): Wei YangVarious virtual personal assistant (VPA) services, e.g. Amazon Alexa and Google Assistant, have become increasingly popular in recent years. This can be partly attributed to a flourishing ecosystem centered around them. Third-party developers are enabled to create VPA applications (or \emph{VPA apps} for short), e.g. Amazon Alexa skills and Google Assistant Actions, which then are released to app stores and become easily accessible by end users through their smart devices.
Similar to their mobile counterparts, VPA apps are accompanied by a privacy policy document that informs users of their data collection, use, retention and sharing practices. The privacy policies are legal documents, which are usually lengthy and complex, hence making it difficult for users to comprehend. Due to this developers may exploit the situation by intentionally or unintentionally failing to comply with them.
In this work, we conduct the first systematic study on the privacy policy compliance issue of VPA apps. We develop \emph{Skipper}, which targets the VPA apps (i.e., \emph{skills}) of Amazon Alexa, the most popular VPA service. \emph{Skipper} automatically depicts the skill into the \emph{declared privacy profile}, by analyzing their privacy policy documents with Natural Language Process (NLP) and machine learning techniques. It then conducts a black-box testing to generate the \emph{behavioral privacy profile} of the skill and checks the consistency between the two profiles. We conduct a large-scale auditing on all 61,505 skills available on Amazon Alexa store. \emph{Skipper} finds that the vast majority of skills suffer from the privacy policy noncompliance issue. Our work reveals the \emph{state quo} of the privacy policy compliance in contemporary VPA apps. Our findings are expected to raise an alert to the app developers and users, and would encourage the VPA app store operators to put in place regulations on privacy policy compliance.