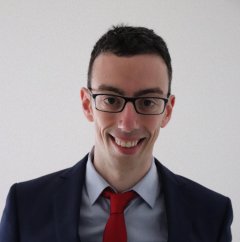
Registered user since Tue 20 Feb 2018
Contributions
View general profile
Registered user since Tue 20 Feb 2018
Contributions
Research Papers
Wed 12 Oct 2022 10:30 - 10:50 at Banquet A - Technical Session 10 - Testing I Chair(s): Gordon FraserVoice-based virtual assistants are becoming increasingly popular. Such systems provide frameworks to developers on which they can build their own apps. End-users can interact with such apps through a Voice User Interface (VUI), which allows to use natural language commands to perform actions. Testing such systems is far from trivial, especially due to the fact that the same command can be expressed using several semantically equivalent utterances, to which the VUI is expected to correctly react. To support developers in testing VUIs, Deep learning (DL)-based tools have been integrated in the development environments to generate paraphrases for selected seed utterances. This is for example the case of the Alexa Developer Console (ADC). Such tools, however, generate a limited number of paraphrases and do not allow to cover several corner cases. In this paper, we introduce VUI-UPSET, a novel approach that aims at adapting chatbot-testing approaches to VUI-testing. Indeed, both systems provide a similar natural-language-based interface to users. We conducted an empirical study to understand how VUI-UPSET compares to existing approaches in terms of (i) correctness of the generated paraphrases, and (ii) capability of revealing bugs. We manually analyzed 5,872 generated paraphrases, totaling 13,310 evaluations. Our results show that the DL-based tool integrated in the ADC generates a significantly higher percentage of meaningful paraphrases compared to VUI-UPSET. However, VUI-UPSET generates a higher number of bug-revealing paraphrases, which allows developers to test more thoroughly their apps at the cost of discarding a higher number of irrelevant paraphrases.
Journal-first Papers
Thu 13 Oct 2022 16:20 - 16:40 at Banquet A - Technical Session 31 - Code Similarities and Refactoring Chair(s): Hua MingRefactoring aims at improving the maintainability of source code without modifying its external behavior. Previous works proposed approaches to recommend refactoring solutions to software developers. The generation of the recommended solutions is guided by metrics acting as proxy for maintainability (e.g., number of code smells removed by the recommended solution). These approaches ignore the impact of the recommended refactorings on other non-functional requirements, such as performance, energy consumption, and so forth. Little is known about the impact of refactoring operations on non-functional requirements other than maintainability. We aim to fill this gap by presenting the largest study to date to investigate the impact of refactoring on software performance, in terms of execution time. We mined the change history of 20 systems that defined performance benchmarks in their repositories, with the goal of identifying commits in which developers implemented refactoring operations impacting code components that are exercised by the performance benchmarks. Through a quantitative and qualitative analysis, we show that refactoring operations can significantly impact the execution time. Indeed, none of the investigated refactoring types can be considered “safe” in ensuring no performance regression. Refactoring types aimed at decomposing complex code entities (e.g., Extract Class/Interface, Extract Method) have higher chances of triggering performance degradation, suggesting their careful consideration when refactoring performance-critical code.
Link to publication DOI Authorizer link